[Speaker Interview] With Yufei Ai, Data Science Manager, Personalisation at Asda
What personalisation strategies have you found most effective in enhancing customer engagement and satisfaction at Asda?
Definitely the ASDA Rewards! By providing personalized offers that are directly tied to a customer's shopping history, we're able to deliver value that is both relevant and timely. We also help support charity with ASDA Rewards. This year the “Cashpot for Schools” received great participation, very positive sentiments from ASDA customers, and of course, raised a lot of fundings for the state schools through UK!
We have observed that customers who participate in the ASDA Rewards program, particularly those who engage with us across multiple channels, exhibit higher retention rates and overall satisfaction with ASDA. This suggests that our personalized approach not only resonates with our customers but also fosters loyalty, as they feel recognized and rewarded for their continued patronage. This data-driven personalization strategy has proven to be a key driver in strengthening our relationship with customers.
How do you leverage data analytics to continuously improve personalisation efforts, and what tools or methodologies have proven most effective in your role?
It's crucial to collect comprehensive data from all customer interaction channels. In my role, leading a data scientist squad, we focus on developing robust data products that integrate and interpret this data to gain insights into customer preferences and shopping behaviors specifically with ASDA.
We build predictive models that analyse past purchase data, browsing patterns, and customer demographics to forecast future shopping behavior and identify products that customers are likely to find appealing. Moreover, we place a strong emphasis on collecting customer feedback through post-purchase engagement and targeted surveys. This feedback is invaluable, as it provides direct insights into the customer experience and helps identify areas for improvement.
Our methodology revolves around transforming actionable insights into data products. This means that our data science work isn't just theoretical; it's converted into practical tools and applications that can be used across the organization to drive personalization strategies. By continuously iterating on these data products and incorporating real-time feedback, we ensure that our personalization efforts are always evolving and improving, keeping pace with customer expectations and market trends.
Can you share an example of a data-driven customer-centric product you developed from prototype to production, and what impact it had on customer experience?
The most recent and significant one was a personalized offer assigning engine. This advanced system was designed with workflows and components that support the assignment of tailored offers to individual customers.
The impact on customer experience was profound. With this engine in place, customers began receiving more consistent and relevant offers, which greatly enhanced their shopping experience and engagement with the brand.
From a business perspective, the development of this engine actually revolutionized our approach to managing offers. It allowed us to translate complex business offer strategies into clear decision logic that could be executed systematically. The engine became a foundational tool for testing innovative methodologies, such as A/B testing and machine learning models, to refine our offer targeting.
Overall, this product not only improved customer satisfaction but also drove business efficiency and adaptability in a rapidly changing retail landscape.
What challenges do you typically face when transitioning data-driven products from prototype to production, and how do you address these challenges?
Transitioning data-driven products from prototype to production often presents several challenges. To address these, we implement a few strategic approaches:
MVP Use Case for Testing: We start with a minimum viable product (MVP) use case that allows us to test the prototype effectively. For instance, we might use post-campaign analysis as a proof of concept (PoC) to estimate the impact of the new prototype. Collaborating with business teams, we plan and execute this use case, gathering feedback and performance data to refine the product before full-scale production.
Engineering Support for Productionisation: Given the novelty of these data products, there is often a knowledge gap. Many team members may not be familiar with the operation and intricacies of the product. To overcome this, the data scientists in my team take a hands-on approach to production work, ensuring that the process is straightforward and adheres to platform policies. We also work closely with engineering teams, fostering a collaborative environment where knowledge and skills are shared, and the product is effectively transitioned into a production-ready state.
Coordination Between Teams: Effective coordination among various teams is crucial during production. This entails aligning stakeholders and analysts on the launch of use cases, communication strategies for targeted customers, and the execution of measurement and analysis following the testing campaigns. It often requires more teamwork than initially anticipated to successfully launch a campaign, especially while approaching Christmas holiday season, but through careful planning and communication, these hurdles can be managed.
Adapting Ways of Working: As organizations become more data-driven, there is an inevitable shift in ways of working. The introduction of a new data product can disrupt established processes, so it's essential to support stakeholders through this transition. We ensure that the positive effects of the product are highlighted and provide the necessary training and support to stakeholders. This helps to scale up delivery, sustain the testing of new ideas and methodologies, and maintain the momentum of becoming a more data-driven organization. By addressing these challenges, generally the whole team needs clear strategies and a collaborative mindset
What conversations are you most looking forward to at the Personalisation Summit?
I'm particularly excited about engaging in conversations that delve into how different companies discover and utilize data products to enhance their personalization efforts. I'm eager to hear about their experiences in building bespoke data products that drive personalization and to exchange insights on the successes and challenges faced during this process.
Additionally, I'm looking forward to discussions around the innovative applications of Generative AI (GenAI) in personalization. I'm interested in exploring ideas on how GenAI can be leveraged reliably to support and scale personalization strategies, ensuring that these advanced technologies are harnessed to deliver meaningful and contextually relevant experiences to customers.
Join Yufei and other industry leaders at the Personalisation Summit this November to explore cutting-edge strategies, share insights, and discover how leading brands are leveraging data-driven personalisation to transform customer experiences and drive business growth.
Download the event agenda here. Don’t miss the chance to network with industry experts and gain actionable insights that will help your organisation stay ahead in today’s competitive landscape!
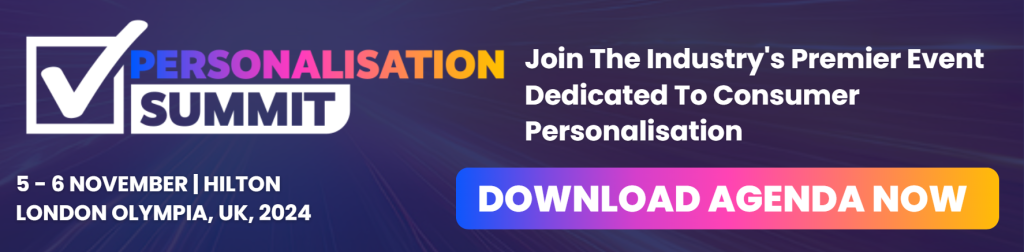
Return to Home